Build, Train, and Fine-Tune Deep Neural Network Architectures for NLP with Python

Natural language processing (NLP) is a subfield of artificial intelligence (AI) that deals with the interaction between computers and human (natural) languages. NLP enables computers to understand, interpret, and generate human language, which has a wide range of applications, including machine translation, text summarization, question answering, and chatbots.
4.6 out of 5
Language | : | English |
File size | : | 14250 KB |
Text-to-Speech | : | Enabled |
Screen Reader | : | Supported |
Enhanced typesetting | : | Enabled |
Print length | : | 564 pages |
Deep neural networks (DNNs) are a type of machine learning model that has been shown to be very effective for NLP tasks. DNNs are able to learn complex relationships between words and phrases, and they can be used to build models that can understand the meaning of text and generate natural-sounding text.
In this article, we will provide a comprehensive guide to building, training, and fine-tuning DNN architectures for NLP tasks in Python. We will cover the following topics:
- An overview of DNN architectures for NLP
- How to build a DNN architecture for NLP
- How to train a DNN architecture for NLP
- How to fine-tune a DNN architecture for NLP
An Overview of DNN Architectures for NLP
There are a variety of different DNN architectures that can be used for NLP tasks. The most common type of DNN architecture for NLP is the Transformer. Transformers are a type of attention-based model that is able to learn long-range dependencies between words and phrases. Other popular DNN architectures for NLP include recurrent neural networks (RNNs) and convolutional neural networks (CNNs).
The choice of which DNN architecture to use for a particular NLP task depends on a number of factors, including the size of the dataset, the complexity of the task, and the desired accuracy.
How to Build a DNN Architecture for NLP
The first step in building a DNN architecture for NLP is to choose the type of architecture that you want to use. Once you have chosen the type of architecture, you will need to decide on the number of layers and the number of units in each layer. The number of layers and units will depend on the size of the dataset and the complexity of the task.
Once you have decided on the architecture of your DNN, you will need to create the model. You can create a DNN model in Python using the Keras library. Keras is a high-level neural networks API, written in Python, that runs on top of TensorFlow. Keras makes it easy to build and train DNN models.
How to Train a DNN Architecture for NLP
Once you have created your DNN model, you will need to train it on a dataset of labeled data. Labeled data is data that has been annotated with the correct output for each input. For example, if you are training a DNN model to perform sentiment analysis, you would need a dataset of labeled data that contains sentences and their corresponding sentiment labels.
You can train a DNN model in Python using the Keras training API. The Keras training API provides a number of different training methods, including gradient descent and backpropagation. You can also use the Keras training API to track the progress of your training.
How to Fine-Tune a DNN Architecture for NLP
Once you have trained your DNN model, you may want to fine-tune it on a specific dataset. Fine-tuning is a technique that allows you to improve the performance of your model on a specific dataset without having to retrain the entire model from scratch.
You can fine-tune a DNN model in Python using the Keras fine-tuning API. The Keras fine-tuning API allows you to specify which layers of your model you want to fine-tune. You can also specify the learning rate and the number of epochs for your fine-tuning.
In this article, we have provided a comprehensive guide to building, training, and fine-tuning DNN architectures for NLP tasks in Python. We have covered the following topics:
- An overview of DNN architectures for NLP
- How to build a DNN architecture for NLP
- How to train a DNN architecture for NLP
- How to fine-tune a DNN architecture for NLP
We encourage you to experiment with different DNN architectures and training methods to find the best approach for your specific NLP task.
4.6 out of 5
Language | : | English |
File size | : | 14250 KB |
Text-to-Speech | : | Enabled |
Screen Reader | : | Supported |
Enhanced typesetting | : | Enabled |
Print length | : | 564 pages |
Do you want to contribute by writing guest posts on this blog?
Please contact us and send us a resume of previous articles that you have written.
Page
Text
Genre
Reader
Library
Paperback
Newspaper
Paragraph
Sentence
Shelf
Bibliography
Foreword
Synopsis
Manuscript
Scroll
Codex
Tome
Bestseller
Memoir
Reference
Encyclopedia
Dictionary
Thesaurus
Narrator
Character
Card Catalog
Borrowing
Stacks
Archives
Periodicals
Academic
Journals
Rare Books
Special Collections
Interlibrary
Literacy
Reading List
Book Club
Theory
Textbooks
Matthew G Stanard
Dylan Jones
Rimma Mykhailovska
S Enders Wimbush
Christa Klickermann
Emm Barnes Johnstone
Robert Charvin
Dale Lane
Dan Williams
Maggie Pace
Cerridwen Greenleaf
Alexis Mantheakis
Ronald A Beghetto
Paul Reitter
Jenny Griffin
Barbara Browning
Melinda Roth
Allie Pleiter
Stephanie Gangi
Margaret M Keith
Light bulbAdvertise smarter! Our strategic ad space ensures maximum exposure. Reserve your spot today!
- Truman CapoteFollow ·16.1k
- Steven HayesFollow ·4.4k
- John MiltonFollow ·19.2k
- Harvey HughesFollow ·13k
- Fyodor DostoevskyFollow ·19.9k
- Louis HayesFollow ·7k
- Jeff FosterFollow ·6.3k
- Alexandre DumasFollow ·12.3k
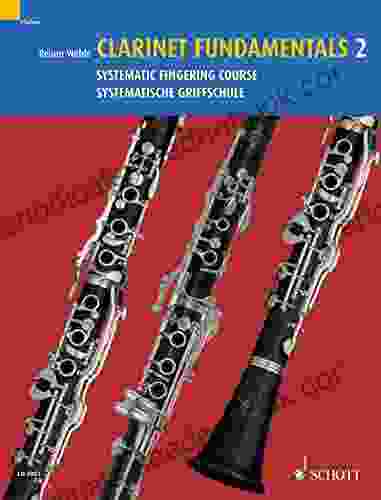

Clarinet Fundamentals: A Systematic Fingering Course for...
Welcome to the exciting world of...
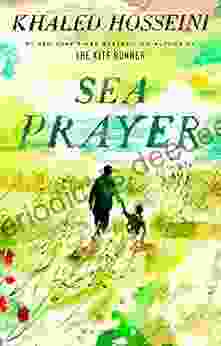

Sea Prayer: A Haunting and Heartbreaking Story of...
Sea Prayer, the latest...
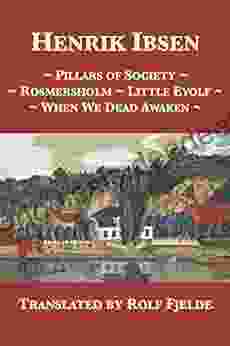

Pillars of Society Rosmersholm Little Eyolf When We Dead...
Henrik Ibsen, the towering...
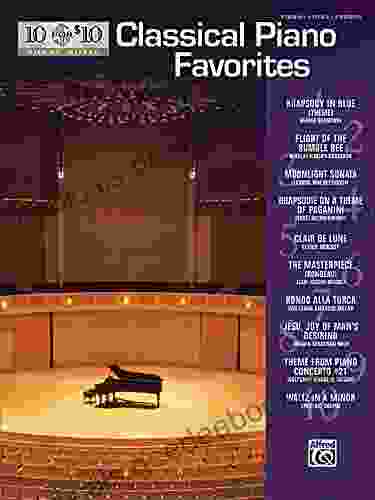

10 For 10 Sheet Music Classical Piano Favorites: A...
Learning to play the...
4.6 out of 5
Language | : | English |
File size | : | 14250 KB |
Text-to-Speech | : | Enabled |
Screen Reader | : | Supported |
Enhanced typesetting | : | Enabled |
Print length | : | 564 pages |