Alzheimer's Disease Classification and Prediction: Harnessing the Power of Machine Learning and Deep Learning

Alzheimer's disease (AD) is a progressive neurological disorder that affects millions of people worldwide. It is characterized by memory loss, cognitive decline, and eventually complete loss of function. As the disease progresses, it can lead to a significant loss of independence and quality of life for both patients and caregivers.
Early detection and diagnosis of AD is essential for managing the disease and improving outcomes. Currently, AD is diagnosed based on clinical assessment and cognitive tests. However, these methods are not always accurate, and they can be subjective.
Machine learning (ML) and deep learning (DL) are powerful artificial intelligence (AI) techniques that have shown great promise in improving the classification and prediction of AD. These techniques can analyze large amounts of data to identify patterns and relationships that are not easily detectable by humans. This allows them to develop more accurate and objective diagnostic models.
4.2 out of 5
Language | : | English |
File size | : | 15560 KB |
Text-to-Speech | : | Enabled |
Screen Reader | : | Supported |
Enhanced typesetting | : | Enabled |
Print length | : | 301 pages |
Lending | : | Enabled |
Paperback | : | 28 pages |
Item Weight | : | 3.2 ounces |
Dimensions | : | 6 x 0.07 x 9 inches |
In this article, we will explore the use of ML and DL in AD classification and prediction. We will discuss the different types of data used for ML and DL models, the different ML and DL algorithms that have been applied to AD, and the challenges and future directions in this field of research.
The data used for ML and DL models in AD classification and prediction can be broadly classified into two types:
- Clinical data: This includes data collected during a patient's clinical assessment, such as medical history, physical examination findings, and cognitive test results.
- Biomarker data: This includes data on biological markers that are associated with AD, such as amyloid-beta plaques, tau tangles, and neurochemical changes in the brain.
Clinical data is typically collected through questionnaires, interviews, and physical examinations. Biomarker data is typically collected through blood tests, cerebrospinal fluid analysis, or brain imaging.
The type of data used for an ML or DL model depends on the specific algorithm being used. Some algorithms can only handle numerical data, while others can handle both numerical and categorical data. Some algorithms require a large amount of data to train, while others can be trained on a smaller dataset.
A variety of ML and DL algorithms have been applied to AD classification and prediction. Some of the most common algorithms include:
- Logistic regression: This is a simple but effective ML algorithm that can be used to classify data into two or more categories. It is often used for AD classification, as it can be trained on a relatively small dataset and it can produce accurate results.
- Support vector machines: This is a more complex ML algorithm that can be used for both classification and regression tasks. It is often used for AD classification, as it can handle high-dimensional data and it is resistant to overfitting.
- Decision trees: This is a simple but powerful ML algorithm that can be used for both classification and regression tasks. It is often used for AD classification, as it is easy to interpret and it can provide insights into the important factors that contribute to AD.
- Random forests: This is an ensemble ML algorithm that combines multiple decision trees to improve accuracy. It is often used for AD classification, as it can handle high-dimensional data and it is resistant to overfitting.
- Convolutional neural networks (CNNs): This is a type of DL algorithm that is specifically designed for processing data that has a grid-like structure, such as images. CNNs have been shown to be very effective for AD classification, as they can learn the complex patterns that are present in brain imaging data.
- Recurrent neural networks (RNNs): This is a type of DL algorithm that is specifically designed for processing sequential data, such as time series data. RNNs have been shown to be effective for AD prediction, as they can learn the temporal patterns that are present in cognitive test data.
Despite the progress that has been made in the use of ML and DL for AD classification and prediction, there are still a number of challenges that need to be addressed. These challenges include:
- Data availability: The amount of data that is available for training ML and DL models is still relatively limited. This can make it difficult to develop models that are accurate and generalizable.
- Data quality: The quality of the data that is available for training ML and DL models can also be a challenge. This can be due to missing data, errors in data collection, or bias in the data.
- Model interpretability: ML and DL models can be complex and difficult to interpret. This can make it difficult to understand how the models make their predictions and to identify the important factors that contribute to AD.
- Clinical translation: The translation of ML and DL models into clinical practice can be challenging. This can be due to regulatory issues, the need for specialized expertise, and the cost of deploying and maintaining models.
Despite these challenges, the future of ML and DL in AD classification and prediction is bright. As more data becomes available, and as ML and DL algorithms continue to improve, we can expect to see more accurate and reliable models that can be used to improve the diagnosis, prognosis, and treatment of AD.
ML and DL are powerful AI techniques that have shown great promise in improving the classification and prediction of AD. These techniques can analyze large amounts of data to identify patterns and relationships that are not easily detectable by humans. This allows them to develop more accurate and objective diagnostic models.
As more data becomes available, and as ML and DL algorithms continue to improve, we can expect to see more accurate and reliable models that can be used to improve the diagnosis, prognosis, and treatment of AD.
4.2 out of 5
Language | : | English |
File size | : | 15560 KB |
Text-to-Speech | : | Enabled |
Screen Reader | : | Supported |
Enhanced typesetting | : | Enabled |
Print length | : | 301 pages |
Lending | : | Enabled |
Paperback | : | 28 pages |
Item Weight | : | 3.2 ounces |
Dimensions | : | 6 x 0.07 x 9 inches |
Do you want to contribute by writing guest posts on this blog?
Please contact us and send us a resume of previous articles that you have written.
Book
Text
Genre
Magazine
Newspaper
Sentence
Bookmark
Glossary
Foreword
Footnote
Scroll
Tome
Classics
Library card
Autobiography
Memoir
Reference
Dictionary
Narrator
Catalog
Archives
Periodicals
Study
Scholarly
Lending
Academic
Journals
Reading Room
Rare Books
Special Collections
Interlibrary
Literacy
Study Group
Thesis
Dissertation
Storytelling
Reading List
Book Club
Theory
Textbooks
Lara Adrian
Val M Runge
Joan Of Dark A K A Toni Carr
Ronald H Chilcote
Dana Buckmir
Jove Chambers
Mara Parker
Sam Staley
Jenelle Montilone
Denis Rothman
Margaret M Keith
Will Butts
Andrew Dempster
Lindsey Sparks
Jennifer Sucevic
Michelle Malkin
William J Bush
T L Toma
Martina Boone
Joyce Murphy
Light bulbAdvertise smarter! Our strategic ad space ensures maximum exposure. Reserve your spot today!
- Ben HayesFollow ·12.5k
- Anton ChekhovFollow ·11.1k
- Brayden ReedFollow ·5.6k
- Gabriel BlairFollow ·2.9k
- Pete BlairFollow ·16.5k
- Bernard PowellFollow ·10.4k
- Stanley BellFollow ·9.9k
- David Foster WallaceFollow ·6.5k
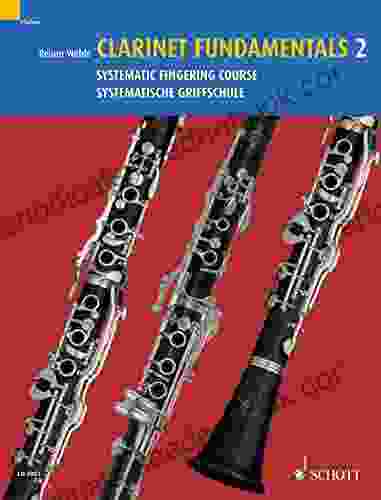

Clarinet Fundamentals: A Systematic Fingering Course for...
Welcome to the exciting world of...
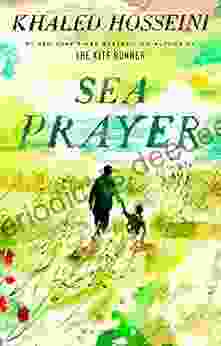

Sea Prayer: A Haunting and Heartbreaking Story of...
Sea Prayer, the latest...
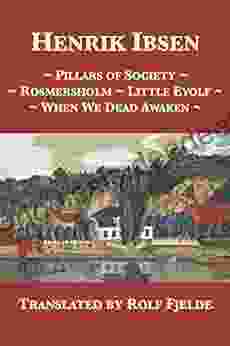

Pillars of Society Rosmersholm Little Eyolf When We Dead...
Henrik Ibsen, the towering...
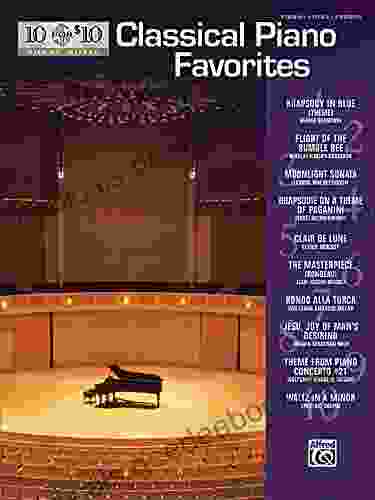

10 For 10 Sheet Music Classical Piano Favorites: A...
Learning to play the...
4.2 out of 5
Language | : | English |
File size | : | 15560 KB |
Text-to-Speech | : | Enabled |
Screen Reader | : | Supported |
Enhanced typesetting | : | Enabled |
Print length | : | 301 pages |
Lending | : | Enabled |
Paperback | : | 28 pages |
Item Weight | : | 3.2 ounces |
Dimensions | : | 6 x 0.07 x 9 inches |